

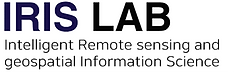

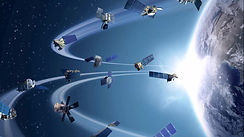
RESEARCH
📚 Representative Works
* Staying the mouse over the screen stops the movement
📝 Publications
Last updated : 2023/03/12
Kang, Y., Jang, E., Im, J., & Kwon, C. (2022). A deep learning model using geostationary satellite data for forest fire detection with reduced detection latency. GIScience & Remote Sensing, 59(1), 2019-2035.
Lee, J., Kim, W., Im, J., Kwon, C., & Kim, S. (2021). Detection of Forest Fire Damage from Sentinel-1 SAR Data through the Synergistic Use of Principal Component Analysis and K-means Clustering. Korean Journal of Remote Sensing, 37(5), 1373-1387.
Sim, S., Kim, W., Lee, J., Kang, Y., Im, J., Kwon, C., & Kim. S. (2020). Wildfire Severity Mapping Using Sentinel Satellite Data Based on Machine Learning Approaches. Korean Journal of Remote Sensing, 36(5), 1109-1123.
Kang, Y., Jang, E., Im, J., Kwon, C., & Kim, S. (2020). Developing a New Hourly Forest Fire Risk Index Based on Catboost in South Korea. Applied Sciences, 10(22), 8213.
Kang, Y., Park, S., Jang, E., Im, J., Kwon, C., & Lee, S. (2019). Spatio-temporal enhancement of forest fire risk index using weather forecast and satellite data in South Korea. Journal of the Korean Association of Geographic Information Studies, 22, 116–130.
Park, S., Son, B., Im, J., Lee, J., Lee, B., & Kwon, C. (2019). Development of Satellite-Based Drought Indices for Assessing Wildfire Risk. Korean Journal of Remote Sensing, 35(6), 1285-1298.
Park, H., Im, J., & Kim, M. (2019). Improvement of satellite-based estimation of gross primary production through optimization of meteorological parameters and high resolution land cover information at regional scale over East Asia. Agricultural and Forest Meteorology, 271, 180-192.
Jang, E., Kang, Y., Im, J., Lee, D. W., Yoon, J., & Kim, S. K. (2019). Detection and monitoring of forest fires using Himawari-8 geostationary satellite data in South Korea. Remote Sensing, 11(3), 271.
Lee, J., Im, J., Kim, K., & Quackenbush, L. J. (2018). Machine learning approaches for estimating forest stand height using plot-based observations and airborne LiDAR data. Forests, 9(5), 268.
Fang, F., Im, J., Lee, J., & Kim, K. (2016). An improved tree crown delineation method based on live crown ratios from airborne LiDAR data. GIScience & Remote Sensing, 53(3), 402-419.
Li, M., Im, J., Quackenbush, L. J., & Liu, T. (2014). Forest biomass and carbon stock quantification using airborne LiDAR data: A case study over Huntington Wildlife Forest in the Adirondack Park. IEEE Journal of Selected Topics in Applied Earth Observations and Remote Sensing, 7(7), 3143-3156.