

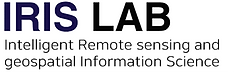

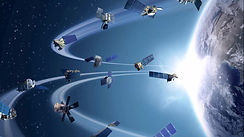
RESEARCH
📚 Representative Works
* Staying the mouse over the screen stops the movement
📝 Publications
Last updated : 2024/10/30
Lee, Y., Cho, D., Im, J., Yoo, C., Lee, J., Ham, Y. G., & Lee, M. I. (2024). Unveiling teleconnection drivers for heatwave prediction in South Korea using explainable artificial intelligence. npj Climate and Atmospheric Science, 7(1), 176.
Cho, D., Im, J., & Jung, S. (2024). A new statistical downscaling approach for short‐term forecasting of summer air temperatures through a fusion of deep learning and spatial interpolation. Quarterly Journal of the Royal Meteorological Society, 150(760), 1222-1242.
Yoo, C., Im, J., Weng, Q., Cho, D., Kang, E., & Shin, Y. (2023). Diurnal urban heat risk assessment using extreme air temperatures and real-time population data in Seoul. Iscience, 26(11).
Lee, S., Yoo, C., Im, J., Cho, D., Lee, Y., & Bae, D. (2023). A hybrid machine learning approach to investigate the changing urban thermal environment by dynamic land cover transformation: A case study of Suwon, republic of Korea. International Journal of Applied Earth Observation and Geoinformation, 122, 103408.
Yoo, C., Im, J., Cho, D., Lee, Y., Bae, D., & Sismanidis, P. (2022). Downscaling MODIS nighttime land surface temperatures in urban areas using ASTER thermal data through local linear forest. International Journal of Applied Earth Observation and Geoinformation, 110, 102827.
Cho, D., Bae, D., Yoo, C., Im, J., Lee, Y., & Lee, S. (2022). All-sky 1 km MODIS land surface temperature reconstruction considering cloud effects based on machine learning. Remote Sensing, 14(8), 1815.
Cho, D., Yoo, C., Son, B., Im, J., Yoon, D., & Cha, D. H. (2022). A novel ensemble learning for post-processing of NWP Model's next-day maximum air temperature forecast in summer using deep learning and statistical approaches. Weather and Climate Extremes, 100410.
Lee, Y., Lee, S., Im, J., & Yoo, C. (2021). Analysis of Surface Urban Heat Island and Land Surface Temperature Using Deep Learning Based Local Climate Zone Classification: A Case Study of Suwon and Daegu, Korea. Korean Journal of Remote Sensing 37(5-3), 1447-1460.
Cho, D., Yoo, C., Im, J., Lee, Y., & Lee, J. (2020). Improvement of spatial interpolation accuracy of daily maximum air temperature in urban areas using a stacking ensemble technique. GIScience & Remote Sensing, 1-17.
Cho, D., Yoo, C., Im, J., & Cha, D. H. (2020). Comparative Assessment of Various Machine Learning‐Based Bias Correction Methods for Numerical Weather Prediction Model Forecasts of Extreme Air Temperatures in Urban Areas. Earth and Space Science, 7(4), e2019EA000740.
Yoo, C., Lee, Y., Cho, D., Im, J., & Han, D. (2020). Improving Local Climate Zone Classification Using Incomplete Building Data and Sentinel 2 Images Based on Convolutional Neural Networks. Remote Sensing, 12(21), 3552.
Yoo, C., Im, J., Park, S., & Cho, D. (2020). Spatial Downscaling of MODIS Land Surface Temperature: Recent Research Trends, Challenges, and Future Directions. Korean Journal of Remote Sensing, 36(4), 609-626.
Yoo, C., Im, J., Cho, D., Yokoya, N., Xia, J., & Bechtel, B. (2020). Estimation of All-Weather 1 km MODIS Land Surface Temperature for Humid Summer Days. Remote Sensing, 12(9), 1398.
Yoo, C., Han, D., Im, J., & Bechtel, B. (2019). Comparison between convolutional neural networks and random forest for local climate zone classification in mega urban areas using Landsat images. ISPRS Journal of Photogrammetry and Remote Sensing, 157, 155-170.
Yoo, C., Park, S., Kim, Y., & Cho, D. (2019). Analysis of Thermal Environment by Urban Expansion using KOMPSAT and Landsat 8: Sejong City. Korean Journal of Remote Sensing, 35(6_4), 1403-1415.
Yoo, C., Im, J., Park, S., & Quackenbush, L. J. (2018). Estimation of daily maximum and minimum air temperatures in urban landscapes using MODIS time series satellite data. ISPRS journal of photogrammetry and remote sensing, 137, 149-162.
Yoo, C., Im, J., Park, S., & Cho, D. (2017). Thermal characteristics of Daegu using land cover data and satellite-derived surface temperature downscaled based on machine learning. Korean Journal of Remote Sensing, 33(6_2), 1101-1118.