

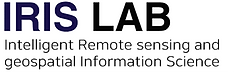

📚 Representative Works
* Staying the mouse over the screen stops the movement
📝 Publications
Last updated : 2024/11/18
Lee, Y., Son, B., Im, J., Zhen, Z., & Quackenbush, L. J. (2024). Two-step carbon storage estimation in urban human settlements using airborne LiDAR and Sentinel-2 data based on machine learning. Urban Forestry & Urban Greening, 94, 128239.
Bae, S., Son, B., Sung, T., Lee, Y., Im, J., & Kang, Y. (2023). Estimation of fractional urban tree canopy cover through machine learning using optical satellite images. Korean Journal of Remote Sensing, 39(5_3), 1009-1029.
Son, B., Lee, Y., & Im, J. (2021). Classification of urban green space using airborne LiDAR and RGB ortho imagery based on deep learning. Journal of the Korean Association of Geographic Information Studies, 24(3), 83-98.