

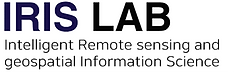

📚 Representative Works
* Staying the mouse over the screen stops the movement
📝 Publications
Last updated : 2024/11/18
Choo, M., Kim, Y., Lee, J., Im, J., & Moon, I. J. (2024). Bridging satellite missions: deep transfer learning for enhanced tropical cyclone intensity estimation. GIScience & Remote Sensing, 61(1), 2325720.
Lee, J., Im, J., & Shin, Y. (2024). Enhancing tropical cyclone intensity forecasting with explainable deep learning integrating satellite observations and numerical model outputs. Iscience, 27(6).
Han, D., Im, J., Shin, Y., & Lee, J. (2023a). Key factors for quantitative precipitation nowcasting using ground weather radar data based on deep learning. Geoscientific Model Development, 16(20), 5895-5914.
Han, D., Choo, M., Im, J., Shin, Y., Lee, J., & Jung, S. (2023b). Precipitation nowcasting using ground radar data and simpler yet better video prediction deep learning. GIScience & Remote Sensing, 60(1), 2203363.
Shin, Y., Lee, J., Im, J., & Sim, S. (2022). An advanced operational approach for tropical cyclone center estimation using geostationary-satellite-based water vapor and infrared channels. Remote Sensing, 14(19), 4800.
Lee, J., Kim, M., Im, J., Han, H., & Han, D. (2021). Pre-trained feature aggregated deep learning-based monitoring of overshooting tops using multi-spectral channels of GeoKompsat-2A advanced meteorological imagery. GIScience & Remote Sensing, 58(7), 1052-1071.
Lee, J., Im, J., Cha, D. H., Park, H., & Sim, S. (2019). Tropical cyclone intensity estimation using multi-dimensional convolutional neural networks from geostationary satellite data. Remote Sensing, 12(1), 108.