

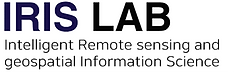

📚 Representative Works
* Staying the mouse over the screen stops the movement
📝 Publications
Last updated : 2021/02/10
Jang, E., Kim, Y., Im, J., & Park, Y. G. (2021). Improvement of SMAP sea surface salinity in river-dominated oceans using machine learning approaches. GIScience & Remote Sensing, 1-23.
Jung, S., Kim, Y., Park, S., & Im, J. (2020). Prediction of Sea Surface Temperature and Detection of Ocean Heat Wave in the South Sea of Korea Using Time-series Deep-learning Approaches. Korean Journal of Remote Sensing., 36(5), 1077-1093.
Jang, E., Im, J., Park, G. H., & Park, Y. G. (2017). Estimation of fugacity of carbon dioxide in the East Sea using in situ measurements and Geostationary Ocean Color Imager satellite data. Remote Sensing, 9(8), 821.
Kwon, Y. S., Jang, E., Im, J., Baek, S. H., Park, Y., & Cho, K. H. (2018). Developing data-driven models for quantifying Cochlodinium polykrikoides using the Geostationary Ocean Color Imager (GOCI). International Journal of Remote Sensing, 39(1), 68-83.
Pyo, J., Ha, S., Pachepsky, Y. A., Lee, H., Ha, R., Nam, G., ... & Cho, K. H. (2016). Chlorophyll-a concentration estimation using three difference bio-optical algorithms, including a correction for the low-concentration range: the case of the Yiam reservoir, Korea. Remote Sensing Letters, 7(5), 407-416.
Jang, E., Im, J., Ha, S., Lee, S., & Park, Y. G. (2016). Estimation of Water Quality Index for Coastal Areas in Korea Using GOCI Satellite Data Based on Machine Learning Approaches. Korean Journal of Remote Sensing, 32(3), 221-234.
Kim, Y. H., Im, J., Ha, H. K., Choi, J. K., & Ha, S. (2014). Machine learning approaches to coastal water quality monitoring using GOCI satellite data. GIScience & Remote Sensing, 51(2), 158-174.